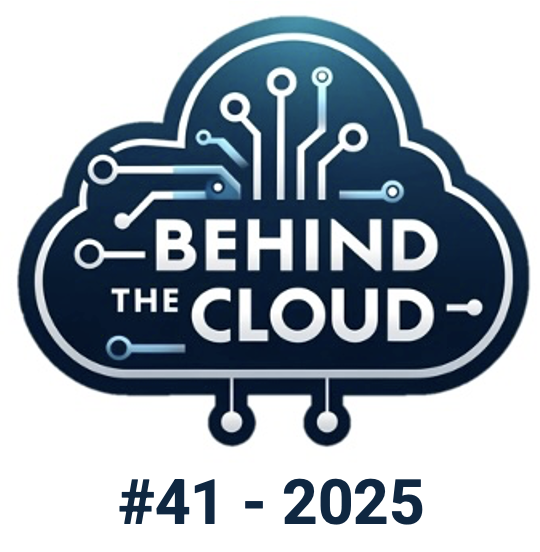
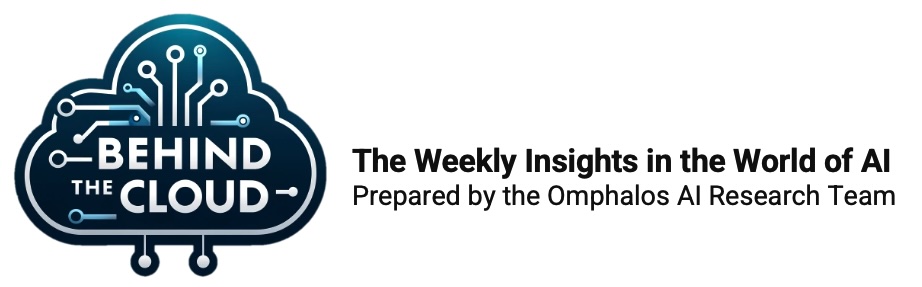
#41 - Behind The Cloud: AI in Risk Management - Navigating Uncertainty in Asset Management (8/9)
The Role of Explainability in Risk Assessment – Building Trust Through Transparency
March 2025
AI in Risk Management: Navigating Uncertainty in Asset Management
This actual series redefines the role of AI in risk management, bridging academic advancements and practical applications in asset management. With a focus on transparency, explainability, and innovation, it will educate both AI enthusiasts and non-specialists about the transformative potential of AI-driven risk strategies.
The Role of Explainability in Risk Assessment – Building Trust Through Transparency
In the world of asset management, trust is a cornerstone of successful client relationships. As Artificial Intelligence (AI) becomes a central tool in managing risks and making investment decisions, the ability to explain how AI models arrive at their conclusions has never been more important. This is where explainability takes center stage.
This chapter explores how explainability enhances risk assessment in AI-driven systems, the challenges involved, and the best practices that asset managers can adopt to build trust and accountability.
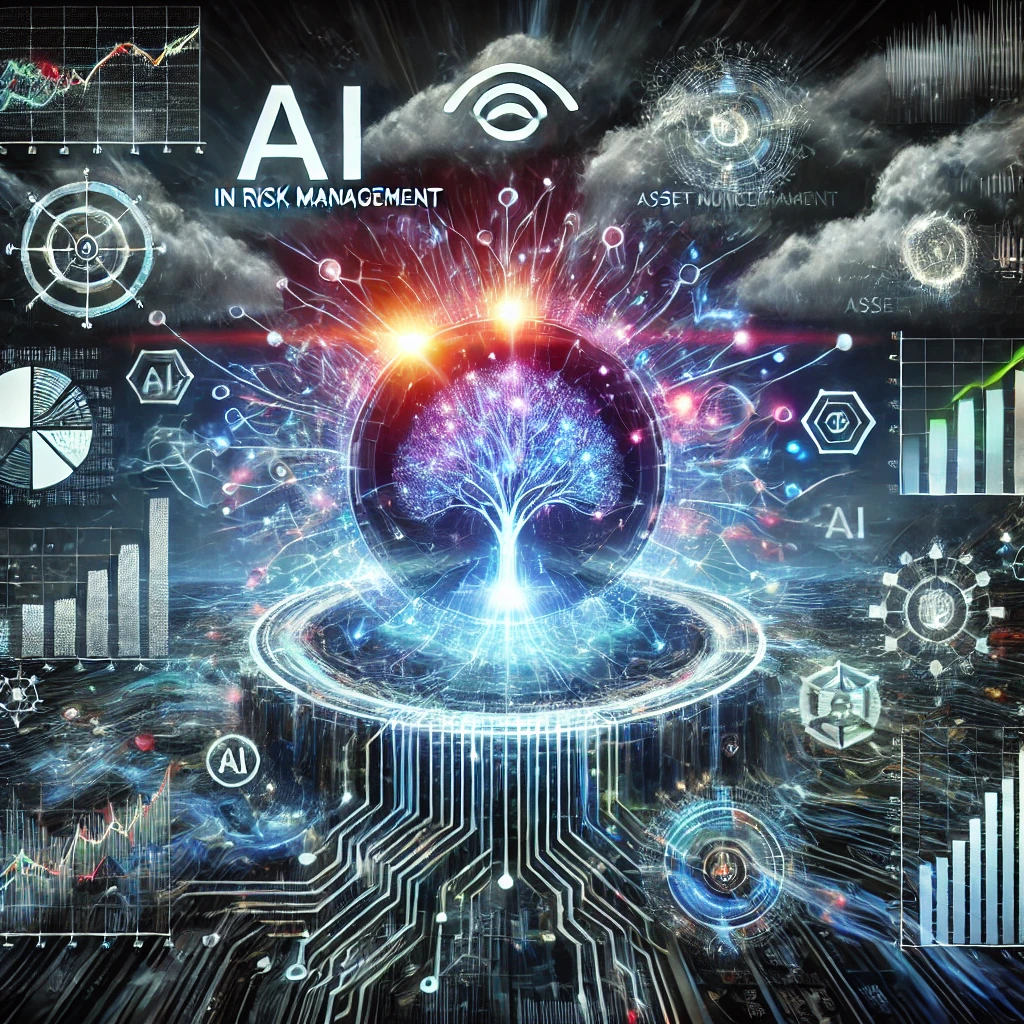
Why Explainability Matters in Risk Assessment
The complexity of modern AI models, such as deep learning networks, often leads to the perception of AI as a “black box”—an inscrutable system whose decisions are difficult to understand. In risk assessment, where decisions carry significant financial and regulatory implications, this lack of transparency can undermine trust and confidence among stakeholders.
Key Drivers of Explainability
- Investor Confidence: Clear explanations of AI-driven decisions help build trust with clients, showing them that strategies are grounded in logic and data.
- Regulatory Compliance: Many jurisdictions require transparency in decision-making, especially for systems used in financial services.
- Error Detection: Explainability enables teams to identify and address errors or biases in AI models before they lead to costly mistakes.
- Alignment with Ethical Standards: Transparent AI ensures that models align with ethical principles, avoiding unintended discrimination or unfair practices.
How Explainability Works in AI Risk Assessment
Explainability focuses on demystifying how AI models process inputs and generate outputs. In risk assessment, this means breaking down complex AI decisions into understandable components. However, it’s important to acknowledge that with highly complex systems—such as deep neural networks—full explainability is not always achievable. While these models provide superior predictive power, their layered architectures often operate as a “black box,” where it is difficult to fully unravel every decision pathway.
Key Techniques for Explainability
- Feature Attribution: Techniques like SHAP (SHapley Additive exPlanations) and LIME (Local Interpretable Model-Agnostic Explanations) reveal the contribution of individual features (e.g., interest rates, market volatility) to the model’s predictions.
- Decision Pathways: While we can map and visualize the decision pathways for AI-based investment strategies, this level of transparency is not fully achievable for complex forecasting models. In those cases, the model’s internal processes remain more opaque, even if the outcomes are interpretable at the strategy level.
- Simplified Models: In some cases, simpler models, such as decision trees, are used alongside complex AI systems to approximate and explain their behavior.
- Natural Language Explanations: AI systems increasingly generate human-readable explanations that describe their decisions in plain language.
Applications of Explainability in Asset Management
Explainability is not just a technical requirement—it is a strategic asset that enhances the effectiveness and reliability of risk assessment processes.
Key Applications Include
- Portfolio Risk Analysis: Transparent AI models allow portfolio managers to understand which factors are driving portfolio risk, helping them make informed adjustments.
- Regulatory Reporting: Explainability tools ensure that firms can provide detailed, understandable reports to regulators about how AI systems assess and manage risks.
- Client Communication: Transparent AI enables firms to clearly articulate risk strategies to clients, fostering trust and improving client retention.
- Bias Detection: Explainability helps firms identify and correct biases in AI systems, ensuring fair and equitable risk assessments.
Challenges in Achieving Explainability
While explainability is critical, achieving it in complex AI systems is not without its challenges.
- Model Complexity: Advanced AI models, like deep neural networks, involve millions of parameters, making their inner workings inherently difficult to explain.
- Trade-Offs with Accuracy: Simplifying models to enhance explainability can sometimes reduce their predictive power, creating a tension between transparency and performance.
- Evolving Regulations: Staying ahead of explainability requirements in different jurisdictions adds another layer of complexity for global asset managers.
- Communication Gap: Bridging the gap between technical explanations and stakeholder understanding requires careful framing and clear communication.
Best Practices for Explainable AI in Asset Management
To ensure that AI-driven risk assessment systems remain transparent and trustworthy, asset managers can adopt the following best practices:
- Integrate Explainability Tools: Use advanced tools like SHAP and LIME to provide clear insights into model behavior.
- Build Expertise in Teams: Train teams to interpret and communicate AI-driven insights effectively, ensuring that stakeholders understand the rationale behind decisions.
- Adopt a Hybrid Approach: Combine explainable models with human expertise to enhance both transparency and decision quality.
- Regular Audits: Conduct periodic reviews of AI systems to ensure their outputs remain consistent, and aligned with goals.
- Ongoing Testing and Validation: Implement systematic testing and validation of AI models to detect model drift, bias, or performance deterioration, ensuring that models remain robust and aligned with risk management objectives over time.
Omphalos Fund: Pioneering Explainable AI in Risk Assessment
At Omphalos Fund, we recognize that explainability is not just a technical feature—it is a fundamental requirement for building trust and accountability in AI systems.
Our Approach to Explainable AI
- Comprehensive Feature Analysis: We use state-of-the-art tools to identify the most significant factors influencing our AI models’ predictions.
- Client Education and Engagement: We actively work to educate our clients on AI, explaining fundamental concepts, sharing insights from cutting-edge research and conferences, and hosting dedicated workshops to deepen their understanding of how AI supports our risk assessment and investment strategies.
- Continuous Improvement: We conduct regular audits to refine our models and ensure they remain transparent, accurate, and aligned with evolving market conditions.
- Collaborative Oversight: Our investment and risk teams work closely with AI engineers to ensure that our models balance accuracy with explainability.
By integrating explainability into every stage of our risk assessment process, we deliver solutions that inspire confidence and drive smarter decision-making.
Conclusion: Transparency as a Catalyst for Trust
In an industry where trust is paramount, explainability is more than a technical challenge—it is a strategic necessity. By making AI systems transparent and understandable, asset managers can build stronger relationships with clients, meet regulatory requirements, and ensure ethical alignment.
At Omphalos Fund, we care deeply about explainability—even though we recognize that not every aspect of large, deep learning models can be fully explained. Our focus is on making AI systems as transparent as possible while ensuring their reliability and effectiveness.
By demystifying AI systems, we empower our clients with the confidence to navigate uncertainty and seize opportunities.
This concludes our 7th chapter in the series “AI in Risk Management: Navigating Uncertainty in Asset Management”.
Next week in “Behind The Cloud”, we’ll wrap up our series with “The Future of Risk Management: AI as the Navigator,” exploring how AI will shape the next generation of risk management systems and redefine the asset management landscape.
Stay tuned!
If you missed our former editions of “Behind The Cloud”, please check out our BLOG.
© The Omphalos AI Research Team – March 2025
If you would like to use our content please contact press@omphalosfund.com