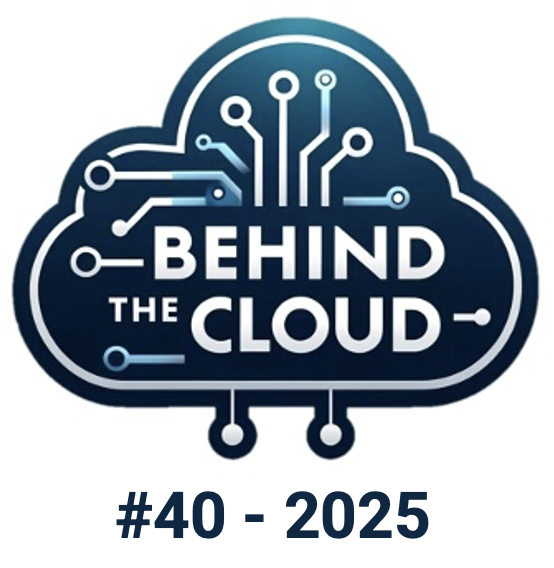
Integrating Risk into AI-Powered Investment Strategies – A Holistic Approach
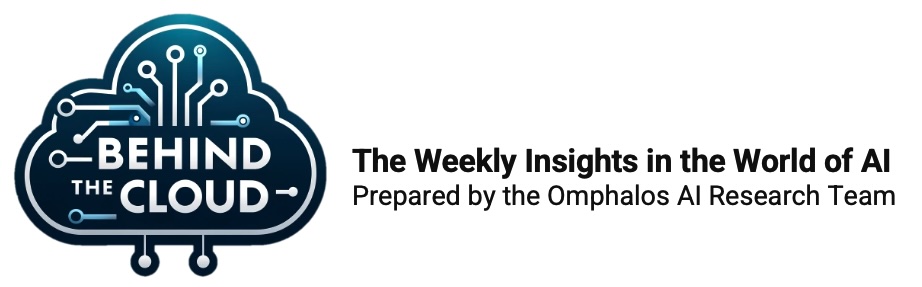
#40 - Behind The Cloud: AI in Risk Management - Navigating Uncertainty in Asset Management (7/9)
Integrating Risk into AI-Powered Investment Strategies – A Holistic Approach
March 2025
AI in Risk Management: Navigating Uncertainty in Asset Management
This actual series redefines the role of AI in risk management, bridging academic advancements and practical applications in asset management. With a focus on transparency, explainability, and innovation, it will educate both AI enthusiasts and non-specialists about the transformative potential of AI-driven risk strategies.
Integrating Risk into AI-Powered Investment Strategies – A Holistic Approach
In the fast-paced world of asset management, risk is an unavoidable companion to opportunity. Balancing the two is critical to creating investment strategies that deliver consistent, long-term returns. Artificial Intelligence (AI) has revolutionized this balancing act by embedding risk management directly into the investment decision-making process.
This chapter delves into how AI integrates risk management into investment strategies, enhancing both precision and resilience in a volatile market landscape.
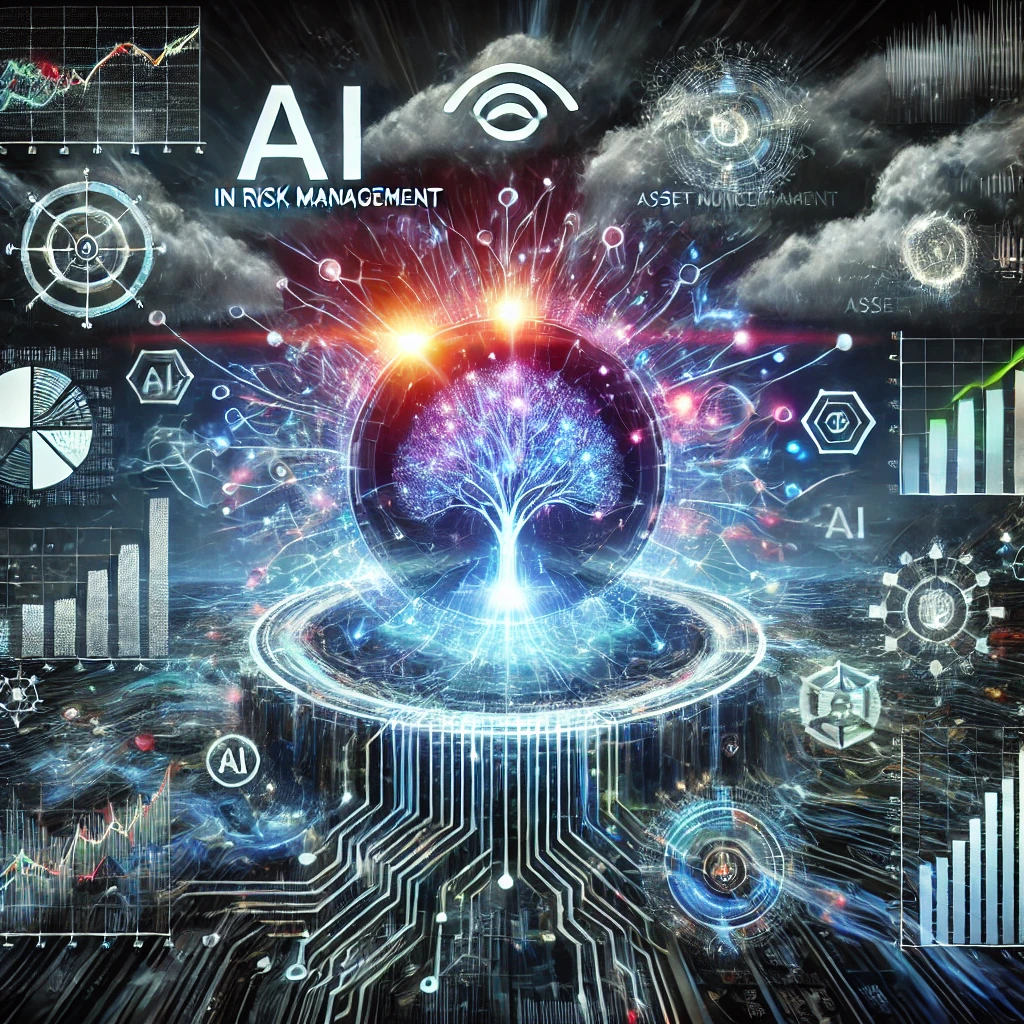
Risk as the Cornerstone of Investment Strategies
Effective investment strategies are not just about maximizing returns; they also focus on minimizing risk. For asset managers, this requires a deep understanding of various risk dimensions, including:
- Market Risk: Fluctuations in asset prices due to market conditions.
- Credit Risk: The potential for loss due to a borrower’s failure to meet financial obligations.
- Liquidity Risk: Challenges in buying or selling assets without impacting their price.
- Operational Risk: Failures in internal processes, systems, or policies.
Incorporating these risk factors into investment strategies is vital for maintaining portfolio stability and meeting client expectations.
How AI Integrates Risk into Investment Strategies
AI enhances risk management by analyzing vast amounts of data, identifying patterns, and providing actionable insights. Here’s how AI works to integrate risk into the heart of investment decision-making:
1. Real-Time Risk Assessment
AI systems continuously monitor market conditions, economic indicators, and geopolitical events to assess risks in real time.
- Example: Identifying increased volatility in the bond market due to unexpected changes in interest rates.
2. Portfolio Optimization with Risk Constraints
Advanced algorithms balance risk and return by optimizing portfolio allocations based on predefined risk tolerance levels.
- Example: Adjusting asset weights in response to increased correlation between equity markets during a downturn.
3. Stress Testing and Scenario Analysis
AI simulates various market conditions, such as recessions or policy changes, to evaluate how a portfolio might perform under stress.
- Example: Assessing the potential impact of a central bank’s monetary policy decision on fixed-income investments.
4. Early Warning Systems
Machine learning models analyze historical data to predict potential risks, enabling proactive risk mitigation.
- Example: Flagging credit risk in a corporate bond due to deteriorating financial ratios or industry trends.
5. AI-Driven Hedging Strategies
AI models identify optimal hedging instruments, such as options, futures, or swaps, to mitigate downside risks without limiting upside potential.
- Example: Constructing an AI-optimized portfolio that includes derivatives to hedge equity exposure while maintaining participation in market gains.
6. Dynamic Rebalancing
AI dynamically adjusts portfolio allocations in response to changing risk factors, ensuring alignment with investment objectives.
- Example: Increasing cash holdings when market volatility spikes to reduce exposure.
Applications in Asset Management
By integrating risk management into investment strategies, AI provides asset managers with the tools to navigate uncertainty and capitalize on opportunities.
Key Applications Include:
- Enhanced Risk-Adjusted Returns: AI ensures that portfolios are optimized not just for returns but also for stability, improving overall risk-adjusted performance.
- Tailored Client Solutions: AI models can incorporate individual client risk tolerances, delivering customized investment strategies.
- Proactive Crisis Management: By anticipating market disruptions, AI helps firms take preemptive measures to protect portfolios.
- Regulatory Alignment: AI-driven strategies ensure compliance with risk-related regulatory requirements, reducing the likelihood of penalties.
Challenges in AI-Integrated Risk Management
Despite its potential, integrating risk into AI-powered strategies comes with its own set of challenges:
- Data Overload: Managing and interpreting vast amounts of data without overwhelming systems or decision-makers.
- Model Transparency: Ensuring that AI models are explainable and that risk factors are clearly understood by stakeholders.
- Evolving Market Dynamics: Adapting AI models to account for new and unforeseen risks in a constantly changing market environment.
- Unforeseen Risks: AI models, especially those trained on historical data, may fail to capture risks that have never occurred before or are outside the model’s experience, leaving blind spots in the strategy.
- Bias in Risk Assessments: Avoiding unintended biases in AI models that could lead to misjudged risk profiles.
- Structural Model Risk: AI models rely on specific assumptions about risk-return dynamics, which might break down in extreme market conditions, requiring continuous adaptation.
Omphalos Fund: Risk-Integrated AI Strategies
At Omphalos Fund, risk is not an afterthought—it is embedded into every step of the investment process through AI-driven analytics and execution strategies.
Our AI-powered risk integration includes:
- AI-Augmented Portfolio Construction: Our models optimize portfolios by balancing risk-adjusted returns and incorporating non-linear dependencies between assets.
- Multi-Agent Market Simulation: We deploy AI-driven multi-agent systems that stress-test portfolios under simulated market conditions, anticipating vulnerabilities before they materialize.
- Tailored Risk Exposure Management: By analyzing macro and microeconomic indicators, our AI models proactively adjust risk exposure in response to shifting market conditions.
- Derivatives-Based Risk Hedging: We construct sophisticated hedging structures, leveraging AI to determine when and how to use options, volatility futures, or credit hedges.
This proactive approach enables us to navigate market uncertainties with confidence, delivering value and security to our clients.
Conclusion: AI as a Strategic Partner in Risk Management
By embedding risk management into investment strategies, AI transforms it from a reactive process into a proactive one. With capabilities ranging from real-time assessments to dynamic rebalancing, AI ensures that risk is not just managed but strategically integrated into every investment decision.
At Omphalos Fund, we view AI as a strategic partner that enhances our ability to deliver risk-adjusted returns, build resilient portfolios, and foster long-term client trust. By embracing AI-powered risk integration, we are shaping a smarter, more secure future for asset management.
AI’s ability to model multi-agent market interactions further strengthens risk-aware decision-making. By simulating how different market participants react under stress conditions, AI allows us to anticipate liquidity shocks, contagion risks, and shifts in volatility regimes before they happen.
This concludes our 7th chapter in the series “AI in Risk Management: Navigating Uncertainty in Asset Management”.
Next week in “Behind The Cloud”, we’ll explore “The Role of Explainability in Risk Assessment,” examining how transparency and accountability ensure that AI-driven risk models remain reliable and trustworthy.
Stay tuned!
If you missed our former editions of “Behind The Cloud”, please check out our BLOG.
© The Omphalos AI Research Team – March 2025
If you would like to use our content please contact press@omphalosfund.com